Tailored and Trending: Key learnings from 3 years of news recommendations
Dr. Christian Leschinski
Every day, we engage with news, and more often, these are curated by recommendation engines. Building such an algorithm poses some unique challenges, different from movie or product recommendations: articles have a short lifetime because nothing is older than yesterday's news. The data is heavily biased by the different positioning of articles on the page, and journalistic principles and brand identity should be represented in the article selection. At Axel Springer National Media and Tech, we overcome these challenges by leveraging our domain knowledge combined with simple statistics instead of black-box machine learning models. This talk will share some of our learnings that can be applied to recommendation systems and data science projects in general.
Dr. Christian Leschinski
Affiliation: Axel Springer National Media and Tech
Dr. Christian Leschinski leads the data science team and the Customer Intelligence team at Axel Springer National Media and Tech. His work is dedicated to build data and AI products that improve the user experience and the monetisation of digital media products and to help organisations to make data-informed decisions. This encompasses use cases ranging from programmatic advertising and subscription pricing to customer analytics and news recommendation.
visit the speaker at: Homepage
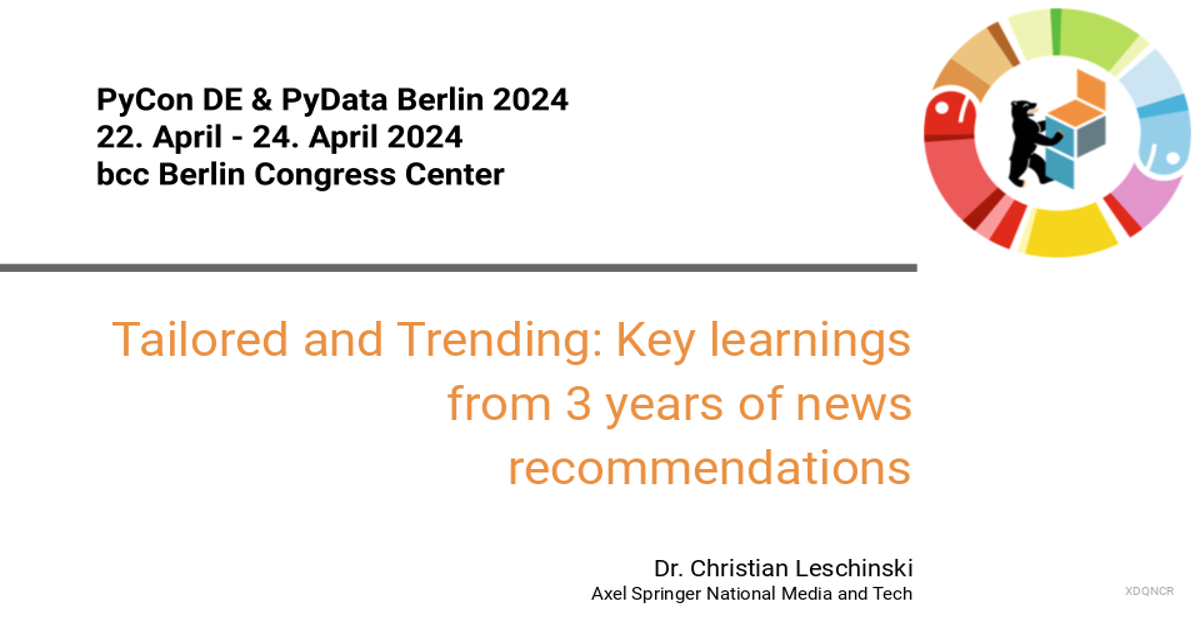