Everything you need to know about change-point detection
Charles Truong
Change-point detection is a crucial processing step when dealing with long and non-stationary time series. It has been applied in many contexts, such as human activity recognition, speech/sound processing and industrial monitoring. This talk guides data scientists, engineers and researchers through the mathematical foundations of this subject, introduces the ruptures Python package for change-point detection, and illustrates algorithms in a biomedical context. By the end, the audience will be able to integrate them into complex data pipelines.
Charles Truong
Affiliation: Paris-Saclay University
Charles Truong is a researcher at Centre Borelli, ENS Paris-Saclay, France. His research interests lie between signal processing, statistics and machine learning. Most of his work is applied in biomedical and industrial contexts. He is the core developper of ruptures, a Python package dedicated to change-point detection algorithms.
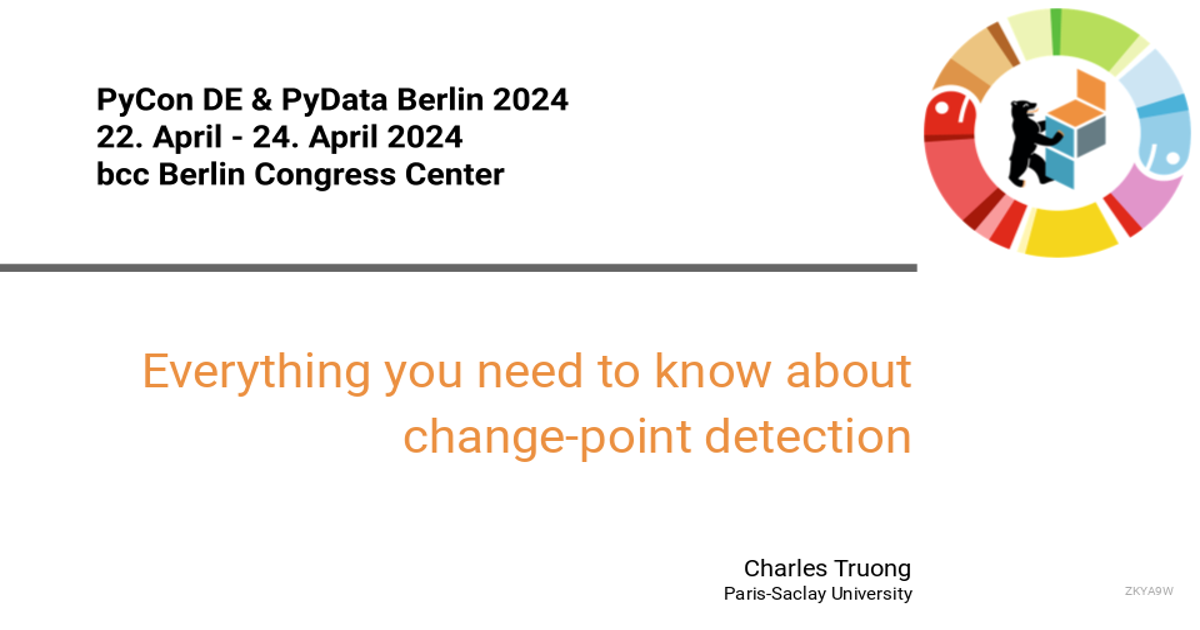