Time series anomaly detection with a human-in-the-loop
Philipp Millet
In the cross-industry wide trend towards industry 4.0 solutions, the amount of gathered sensor data is ever growing. Through the sheer amount of data, manual or human-based monitoring of the collected time series data becomes cumbersome if not even impossible. Yet, careful inspection of the time series data and identification of possible anomalies therein is crucial to detect problems in the underlying processes. To resolve this demand, ZEISS is developing a fully automated time series processing tool that performs ML based time series anomaly detection with a human-in-the-loop.
Philipp Millet
Affiliation: ZEISS Digital Partners
With a background in particle physics, Philipp Millet has been working as Data Scientist for HotSprings/Umlaut/Accenture in various projects and domains. In 2023 he joined ZEISS Digital Partners as a Machine Learning Engineer. His focus is getting Data Science projects from a PoC stage into production.
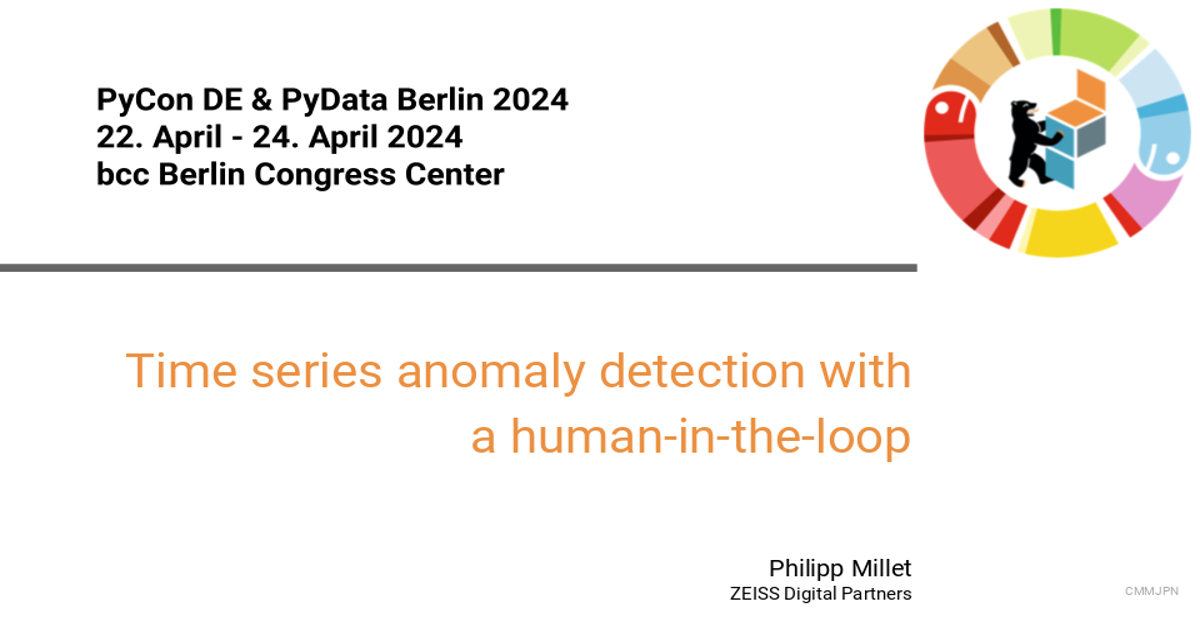