Flix CitySnap: How we use GenAI and not only to collect captivating images for cities and confirm their locations
Andrei Chernov
In recent years, text-to-text models like ChatGPT and text-to-image models such as DALL-E 3 have become increasingly integrated into various industries. The main aim of these initiatives is typically to generate text or images. In our presentation, we propose a slightly different approach to leveraging these models commercially. Our objective is to gather images for thousands of cities that inspire travel. We utilize ChatGPT to tailor prompts for our business requirements, enabling efficient image retrieval through API queries from free stock image services. Then we apply image-to-text models to confirm the images' locations. Finally, we need to adjust the resolution of images for display across various platforms, such as social media campaigns on Instagram, email marketing, and on our website. To achieve this, we have used an automated cropping service to get images in the required aspect ratios, followed by Lanczos sampling for downscaling the images. This integration of cutting-edge models has resulted in an automated, highly flexible process that aligns with varied business needs. The most significant challenge we faced was verifying that an image indeed depicted the target city. We experimented with various models for this purpose and will present our findings. Our approach is cost-efficient; processing several hundred cities amounts to only a few euros, and we have utilized commonly available services, making replication easy for everyone.
Andrei Chernov
Affiliation: Flix
Career: Since 2022, I have continued my career as a data scientist at FlixBus. From 2018 to 2022, I worked as a Data Scientist in banking.
Education: From 2021 to 2022, I received a micro master's degree in Finance. From 2019 to 2021, I received a master's degree in computer science. From 2015 to 2019, I received a bachelor's degree in applied math.
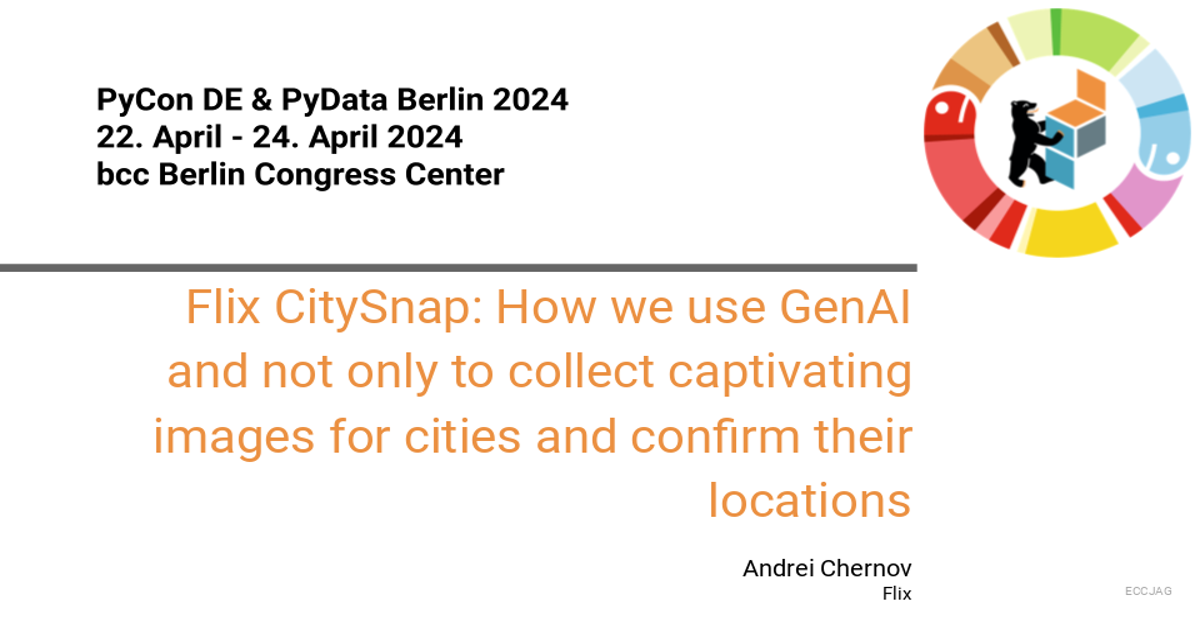