Missing Data, Bayesian Imputation and People Analytics with PyMC
Nathaniel Forde
We demonstrate a range of different approaches to missing data imputation in employee engagement survey data. Contrasting frequentist style full-information maximum likelihood approaches with more direct Bayesian imputation and chained equation methods, we highlight how the different assumptions regarding the missing-data license different inferences about the imputed values and ultimately the plausible causal narratives which can be expressed in PyMC. In particular we avail of the hierarchical nature of employee engagement data to justify a hierarchical approach to justifying the (MAR) missing-at-random assumption for imputation schemes in People Analytics.
Nathaniel Forde
Affiliation: Personio
I'm a data scientist from Dublin, working at Personio on a range of revenue or customer focused areas. Previously I worked with CarTrawler on pricing and insurance risk modelling, and with Marsh and McLennan in areas of re-insurance and catastrophic risk. Before this i worked in Paddy Power Betfair on models of risk indicators for gambling as part of a responsible gambling initiative. I''m broadly interested in problems of risk and confounding.
visit the speaker at: Homepage
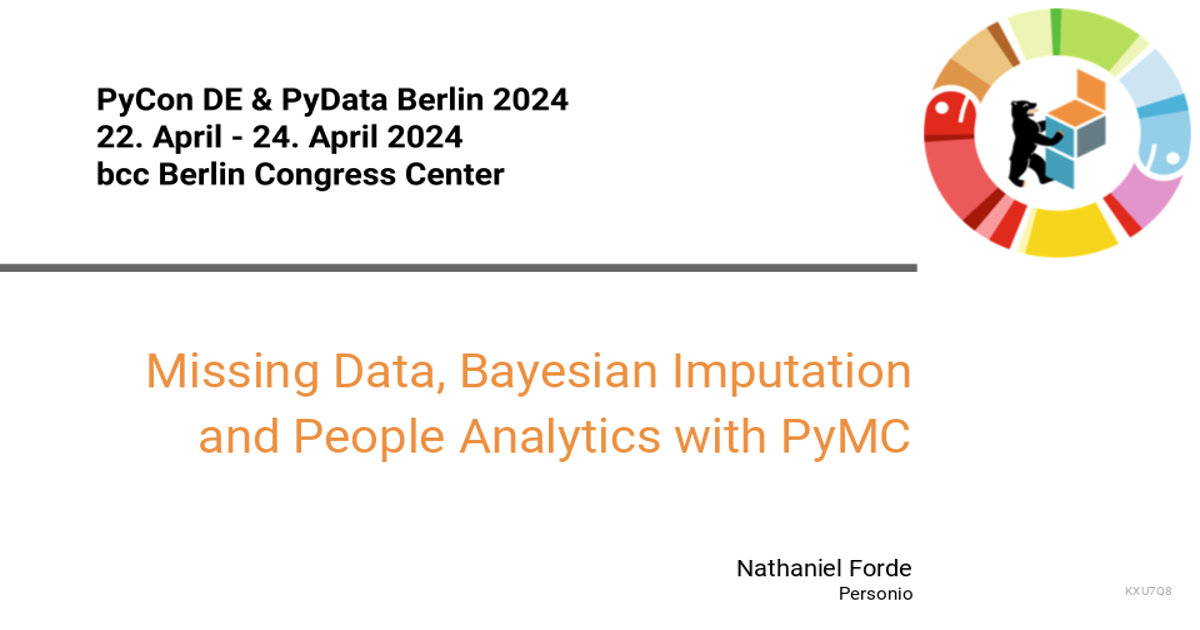