Breaking AI Boundaries: Fairness Metrics in Unstructured Data Domains
Daniel Klitzke
This presentation addresses the rare use of machine learning fairness metrics in domains with indirect human impact, e.g., automotive engineering. We briefly map out the space of use cases to examine the necessity, potential benefits, and challenges of applying fairness-related techniques. The main focus then lies on proposing solutions for overcoming identified hurdles, especially regarding the application in unstructured data domains, such as image and audio recognition and large text document analysis. Our approach includes strategies for detecting key subgroups and providing clear explanations for model failures. We also highlight two open-source tools, Sliceguard and Spotlight, for practical implementation.
Daniel Klitzke
Affiliation: Renumics GmbH
I'm a seasoned AI professional with an additional background in software engineering and web development. Having participated in and led quite a few machine learning-based projects in the engineering domain, I've worked on various ML problems, ranging from ML on images and 3D data to audio and time series analysis. My expertise in software engineering makes it easy for me to bring ML solutions to production. Currently, my focus is on helping people build effective ML models, from planning out projects to creating performant models and productionizing them. I'm particularly passionate about data curation, and I'm excited to be a part of the team building Renumics Spotlight, a free data curation tool.
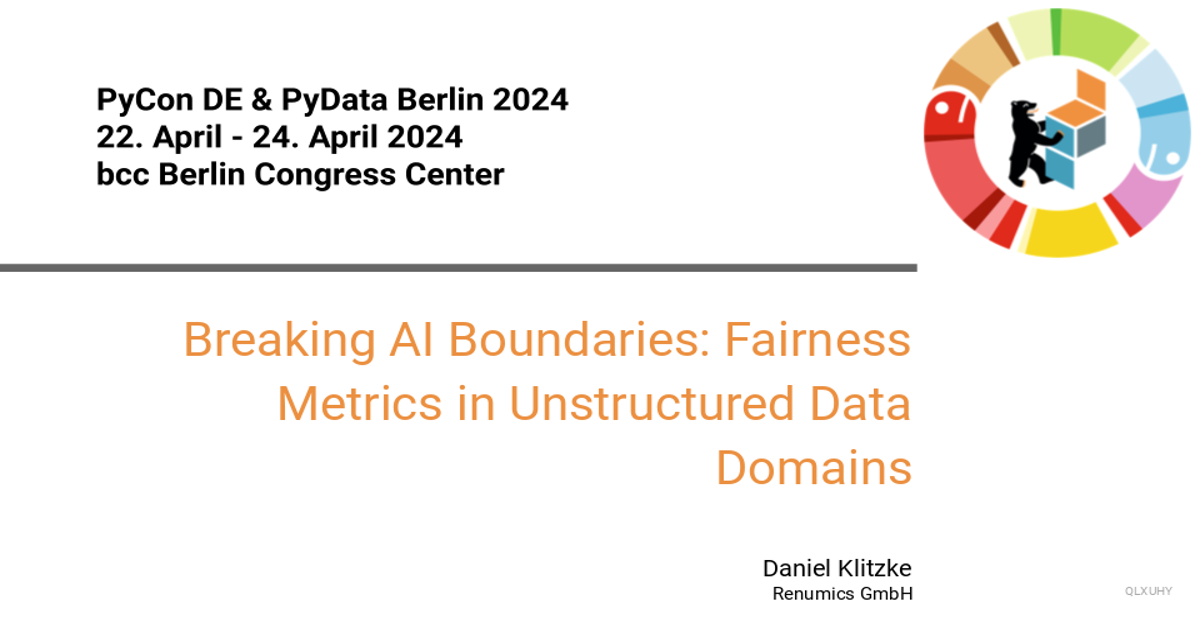